From Data to Decisions: Stuart Frost on the Role of Analytics in Industrial Innovation
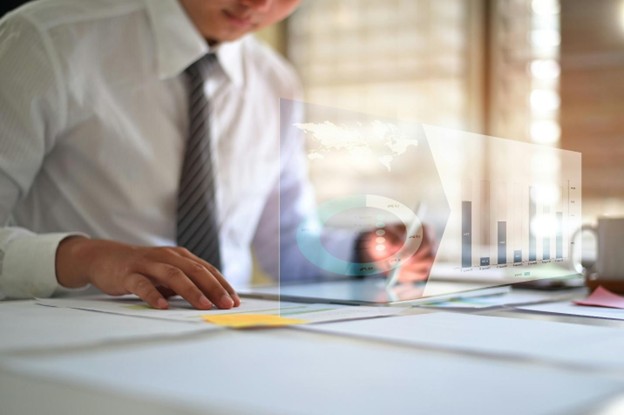
The ability to transform raw data into actionable decisions is redefining industrial progress. Analytics, powered by causal AI, is at the forefront of this shift, offering businesses a deeper understanding of cause-and-effect relationships. Unlike traditional models, which often rely on correlation, causal AI enables industries to predict outcomes with greater accuracy and confidence.
Geminos CEO Stuart Frost, who has established himself as a leader in digital transformation, discusses how such innovation is unlocking efficiencies, reducing risks, and driving smarter strategies, making it an essential tool for modern industrial leaders.
Understanding Causal AI and Its Relevance in the Industry
As industries embrace data-driven decision-making, understanding the difference between correlation and causation has become vital. Businesses that rely on analytics to guide strategy need clarity on what drives change. This is where causal AI comes into play, offering a way to identify the root causes of trends, behaviors, and outcomes. By advancing beyond the limitations of traditional AI, causal AI is transforming how industries operate and adapt.
“Causal AI is an advanced approach to machine learning and analytics that focuses on identifying cause-and-effect relationships,” says Stuart Frost. “Unlike conventional AI systems, which often rely on correlations to make predictions, causal AI establishes the ‘why’ behind patterns.”
It uses data to observe associations and to understand how changing one variable impacts another. This methodology transforms raw data into meaningful insights, allowing businesses to act with precision rather than assumption. At its core, causal AI eliminates the guesswork surrounding correlation.
A standard AI model might detect a pattern between equipment downtime and weather conditions without knowing if one truly causes the other. Causal AI identifies this connection but also determines whether action on weather forecasts could reduce downtime, offering actionable intelligence. This principle enables businesses to simulate interventions and predict outcomes before implementing changes.
While traditional AI has brought significant advancements, its reliance on correlation can lead to missed opportunities or even flawed decisions. Many AI systems process data at scale and uncover relationships within it, but they often fail to account for contextual factors or hidden variables. This makes traditional approaches less suitable for addressing the complexity of real-world industrial settings.
Industrial environments, such as manufacturing plants or energy grids, demand precision and adaptability. These systems are influenced by numerous interconnected factors, from human behavior to equipment variability. Traditional AI models often struggle to account for these dynamics, offering solutions based on surface-level patterns rather than deeper causes. As a result, recommendations might not align with actual operational needs, leading to inefficiencies at best and costly errors at worst.
Causal AI addresses these gaps by providing a clearer understanding of the “cause” behind observed phenomena. When analyzing production delays, traditional AI might point to increased maintenance activity as a key factor. However, causal AI can identify whether the maintenance itself is the true cause or merely a side effect of another variable, like inadequate staff training. By pinpointing the root issue, teams can implement targeted solutions rather than wasting resources on the wrong priorities.
The Role of Analytics in Causal AI
Analytics serves as the backbone for implementing causal AI in industrial innovation. It transforms unstructured data into valuable insights that drive informed decision-making. By focusing on data collection, advanced analysis, and real-time adaptability, analytics empowers businesses to optimize processes, minimize risks, and improve outcomes.
Reliable data collection is the first step in ensuring that analytics can deliver meaningful results. In industrial environments, data streams are generated from numerous sources, including IoT sensors, manufacturing equipment, and operational logs. Capturing this data across various points within the supply chain or production process is critical for creating a complete and accurate picture of operations.
Integration is equally important, as data often exists in silos across different departments and systems. Analytics platforms consolidate this dispersed information, ensuring it is accessible for comprehensive examination. A key factor in successful data collection and integration is maintaining data quality. Inconsistent or incomplete data skews results and limits actionable insights.
Once the data is organized, analytics takes the lead in identifying meaningful patterns and extracting actionable insights. Statistical models and algorithms play a central role at this stage, sifting through complex datasets to highlight relationships and trends. What sets causal AI apart is its ability to extend past recognizing connections. It determines what actually drives changes in outcomes by using causal inference tools.
Notes Frost, “Predictive maintenance in industrial plants depends on understanding which factors contribute to equipment failures.”
Traditional analytics might highlight correlations, such as frequent downtime being linked to older machinery. However, causal analytics aims to uncover whether age is the true cause or if other underlying conditions, like inadequate lubrication or operator errors, play a larger role. By pinpointing root causes, companies can take focused actions that directly prevent future failures.
In industrial settings, the ability to analyze information in real-time is transformative. Downtime, defects, or process inefficiencies can result in costly delays. Analytics enables the constant monitoring of operations through data streams that update continuously. This ensures that businesses can detect issues early and respond immediately.
A common application involves automated production lines equipped with IoT sensors. These sensors send performance data to analytical systems, which assess deviations from expected benchmarks. If an anomaly, such as a temperature spike in machinery, is detected, the system identifies the potential cause and sends alerts. Operators can then address the issue before it escalates into a complete shutdown.
Industrial Applications of Causal AI Powered by Analytics
Causal AI, driven by advanced analytics, is reshaping the way industries operate by providing clarity on the “why” behind critical operations and challenges. Its ability to uncover cause-and-effect relationships allows businesses to make smarter, faster, and more reliable decisions. In industrial settings, this capability transforms efficiency, reliability, and adaptability across several key areas.
As the potential of causal AI in industrial settings becomes evident, so do the challenges and ethical questions that accompany its adoption. From data access issues to ethical quandaries and scalability barriers, businesses must navigate a complex landscape to realize the benefits of causal AI analytics.
The success of causal AI in industrial applications hinges on the availability of high-quality data. However, acquiring reliable and unbiased datasets is far from straightforward. Data sources in industrial environments often exist in silos, scattered across different departments, systems, and even third-party vendors. This fragmentation makes it challenging to gather the comprehensive data required for causal analysis.
Analytics combined with causal AI is fundamentally enhancing how industries address challenges and seize opportunities. By moving from correlation to causation, businesses gain precision in decision-making, reducing inefficiencies while increasing adaptability. This innovation empowers leaders to predict outcomes, optimize operations, and act with confidence in complex environments.
“As industries continue to digitize and data grows in complexity, the role of analytics in causal AI will only expand,” says Frost.
This technology holds the potential to redefine industrial processes, fostering smarter strategies and sustainable growth. Organizations that embrace this paradigm shift are better positioned to shape the future of their sectors and drive lasting innovation.